Multi-level supervision and prediction for geo-distributed, heterogeneous infrastructures in the Cloud/Edge/IoT continuum
— Preview
Define methods, using distributed machine learning in particular, to enable their efficient management, provide means of securing them and ensure a variety of QoS properties.
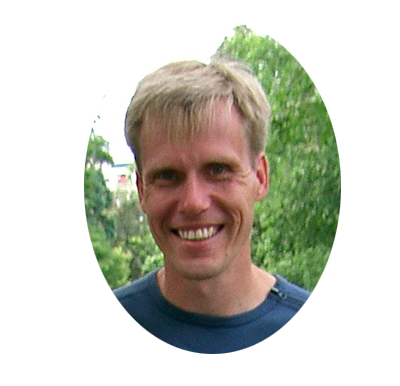
Mario Südholt, Professor IMT
Keywords : Supervision, prediction, mitigation; heterogeneous multi-level infrastructures, applications and software stacks; AI, Cloud/Edge/Internet of Things continuum
Today, the cloud is the main infrastructure on which the world’s largest distributed applications are built. New requirements are emerging for computing and storage capacities at the edge, and for the many Internet of Things (IoT) devices that are part of smart cities, the industry of the future, vehicular networks… The resulting cyber ecosystem, the Cloud-Edge-IoT continuum, is characterized by highly heterogeneous infrastructures as well as multi-layered applications and software stacks. Monitoring infrastructures and applications, detecting anomalies in service and application execution, and predicting resource utilization are fundamental services for the continuum. They help to secure applications and ensure numerous quality-of-service properties. However, growing heterogeneity and the use of increasingly complex software stacks require new methods for developing these services. The SPIREC project will address the challenges of monitoring continuum services, detecting their execution anomalies and predicting their resource utilization.
The partners will also develop software components and tools to integrate these functionalities into existing infrastructures and applications, in particular SLICES and future software ecosystems.
— Missions
— Our researches
— Partners
Consortium
Inria, IMT, CNRS, CEA, Université Versailles
Saint-Quentin en Yvelines, Université de Lorraine
Our teams in France
— Publications
Conference papers
Other projects