Understanding, improving and reducing the environmental impact of cloud computing
— Preview
CARECloud project aims to drastically reduce the environmental impact of cloud infrastructures
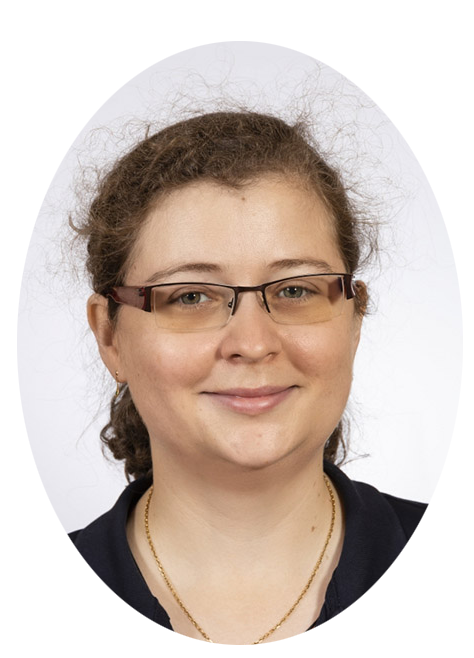
© Jean-Claude MOSCHETTI / CNRS Images
Anne-Cécile Orgerie, CNRS Research Director
Keywords : Cloud computing, environmental impact, energy footprint
Cloud computing offers users considerable computing and storage capacity. The maturity of virtualization techniques has enabled the emergence of complex virtualized infrastructures, capable of rapidly deploying and reconfiguring virtual and elastic resources, in increasingly distributed infrastructures. This transparent resource management gives users the illusion of access to flexible, unlimited and virtually immaterial resources. However, the power consumption of these clouds is very real and a cause for concern, as are their overall greenhouse gas (GHG) emissions and the consumption of critical raw materials used in their manufacture.
At a time when climate change is a growing concern, with serious consequences for people and the planet worldwide, all sectors (transport, construction, agriculture, industry, etc.) must contribute to the effort to reduce GHG emissions. Clouds, despite their ability to optimize processes in other sectors, are no exception to this observation: the increasing slope of their GHG emissions must be reversed, or their potential benefits in other sectors will be wiped out. This is why the CARECloud project aims to drastically reduce the environmental impact of cloud infrastructures.
CARECloud is organized into 4 work packages. Work package 0 is dedicated to project management and scientific mediation.
— Missions
— Our researches
— Partners
Consortium
Inria, CNRS, IMT, Université Toulouse 3 Paul Sabatier, Université Côte d’Azur, Université de Lille, ENS Lyon, Université Claude Bernard Lyon 1, Université Toulouse Jean Jaurès, INSA Toulouse
Our teams in France
— Publications
Journal articles
- Guillaume Fieni, Romain Rouvoy, Lionel Seinturier. xPUE: Extending Power Usage Effectiveness Metrics for Cloud Infrastructures. IEEE Transactions on Sustainable Computing, In press, ⟨10.1109/TSUSC.2025.3549687⟩. ⟨hal-04980997⟩
- Guillaume Fieni, Daniel Romero Acero, Pierre Rust, Romain Rouvoy. PowerAPI: A Python framework for building software-defined power meters. Journal of Open Source Software, 2024, 9 (98), pp.6670. ⟨10.21105/joss.06670⟩. ⟨hal-04601379⟩
- Pierre Jacquet, Thomas Ledoux, Romain Rouvoy. ScroogeVM: Boosting Cloud Resource Utilization with Dynamic Oversubscription. IEEE Transactions on Sustainable Computing, 2024, pp.1-13. ⟨10.1109/TSUSC.2024.3369333⟩. ⟨hal-04466538⟩
Conference papers
- Édouard Guégain, Alexandre Bonvoisin, Mathieu Acher, Clément Quinton, Romain Rouvoy. Exploring Performance of Configurable Software Systems: the JHipster Case Study. EASE 2025 - 29th International Conference on Evaluation and Assessment in Software Engineering, Jun 2025, Istanbul, Turkey. ⟨hal-05003699v2⟩
- Pierre Jacquet, Camille Coti, Marcos Dias de Assuncao, Romain Rouvoy. CINERGY: Reasoning over the Worst Case Power Consumption of Cloud Virtual Machines. CCGRID 2025: International Symposium on Cluster, Cloud and Grid Computing, May 2025, Tromso, Norway, Norway. ⟨hal-04981001⟩
- Édouard Guégain, Rémy Raes, Noé Chachignot, Clément Quinton, Romain Rouvoy. AndroWatts: Unpacking the Power Consumption of Mobile Device's Components. MOBILESoft'25 - 12th International Conference on Mobile Software Engineering and Systems, Apr 2025, Ottawa, Canada. ⟨hal-04928609⟩
- Francesco Diana, Othmane Marfoq, Chuan Xu, Giovanni Neglia, Frédéric Giroire, et al.. Attribute Inference Attacks for Federated Regression Tasks. 39th Annual AAAI Conference on Artificial Intelligence - AAAI 2025, Feb 2025, Philadelphia (Pennsylvania), United States. ⟨hal-04878082⟩
- Emanuele Natale, Davide Ferre’, Giordano Giambartolomei, Frédéric Giroire, Frederik Mallmann-Trenn. On the Sparsity of the Strong Lottery Ticket Hypothesis. NeurIPS 2024 - 38th Conference on Neural Information Processing Systems, Dec 2024, Vancouver, Canada. ⟨hal-04741369v2⟩
- Vladimir Ostapenco, Laurent Lefèvre, Anne-Cécile Orgerie, Benjamin Fichel. Exploring RAPL as a Power Capping Leverage for Power-Constrained Infrastructures. ICA3PP 2024 - 24th International Conference on Algorithms and Architectures for Parallel Processing, Oct 2024, Macau SAR, China. pp.1-10. ⟨hal-04742418⟩
- Pierre Jacquet, Thomas Ledoux, Romain Rouvoy. SLACKVM: Packing Virtual Machines in Oversubscribed Cloud Infrastructures. 2024 CLUSTER - IEEE International Conference on Cluster Computing, Sep 2024, Kobe, Japan. pp.1-12, ⟨10.1109/CLUSTER59578.2024.00024⟩. ⟨hal-04636648⟩
- Danilo Carastan-Santos, Georges da Costa, Millian Poquet, Patricia Stolf, Denis Trystram. Light-weight prediction for improving energy consumption in HPC platforms. Euro-Par 2024, Carretero, J., Shende, S., Garcia-Blas, J., Brandic, I., Olcoz, K., Schreiber, M., Aug 2024, Madrid, Spain. pp.152-165, ⟨10.1007/978-3-031-69577-3_11⟩. ⟨hal-04566184v2⟩
- Tiago da Silva Barros, Davide Ferre, Frederic Giroire, Ramon Aparicio-Pardo, Stephane Perennes. Scheduling Machine Learning Compressible Inference Tasks with Limited Energy Budget. ICPP 2024 - 53rd International Conference on Parallel Processing, Aug 2024, Gotland, Sweden. pp.961 - 970, ⟨10.1145/3673038.3673106⟩. ⟨hal-04676376⟩
- Thibault Simon, David Ekchajzer, Adrien Berthelot, Eric Fourboul, Samuel Rince, et al.. BoaviztAPI: a bottom-up model to assess the environmental impacts of cloud services. HotCarbon'24 - 3rd Workshop on Sustainable Computer Systems, Jul 2024, Santa Cruz, United States. ⟨hal-04621947v3⟩
- Clément Courageux-Sudan, Anne-Cécile Orgerie, Martin Quinson. Studying the end-to-end performance, energy consumption and carbon footprint of fog applications. ISCC 2024 - 29th IEEE Symposium on Computers and Communications, Jun 2024, Paris, France. pp.1-7. ⟨hal-04581677⟩
- Tristan Coignion, Clément Quinton, Romain Rouvoy. A Performance Study of LLM-Generated Code on Leetcode. EASE'24 - 28th International Conference on Evaluation and Assessment in Software Engineering, Jun 2024, Salerno, Italy. ⟨10.1145/3661167.3661221⟩. ⟨hal-04525620⟩
- Wedan Emmanuel Gnibga, Andrew A Chien, Anne Blavette, Anne-Cécile Orgerie. FlexCoolDC: Datacenter Cooling Flexibility for Harmonizing Water, Energy, Carbon, and Cost Trade-offs. e-Energy 2024 - 15th ACM International Conference on Future and Sustainable Energy Systems, Jun 2024, Singapore, Singapore. pp.108-122, ⟨10.1145/3632775.3661936⟩. ⟨hal-04581701⟩
- Tiago da Silva Barros, Frédéric Giroire, Ramon Aparicio-Pardo, Stéphane Perennes, Emanuele Natale. Scheduling Compressible Tasks: Application Neural Network Inference. AlgoTel 2024 – 26èmes Rencontres Francophones sur les Aspects Algorithmiques des Télécommunications, May 2024, Saint-Briac-sur-Mer, France. ⟨hal-04566780⟩
- Tiago da Silva Barros, Frédéric Giroire, Ramon Aparicio-Pardo, Stephane Perennes, Emanuele Natale. Scheduling with Fully Compressible Tasks: Application to Deep Learning Inference with Neural Network Compression. CCGRID 2024 - 24th IEEE/ACM international Symposium on Cluster, Cloud and Internet Computing, IEEE/ACM, May 2024, Philadelphia, United States. ⟨10.1109/CCGrid59990.2024.00045⟩. ⟨hal-04497548⟩
- Pierre Jacquet, Thomas Ledoux, Romain Rouvoy. SweetspotVM: Oversubscribing CPU without Sacrificing VM Performance. CCGrid'24: 24th IEEE/ACM international Symposium on Cluster, Cloud and Internet Computing, May 2024, Philadelphia, United States. pp.1-10, ⟨10.1109/CCGrid59990.2024.00026⟩. ⟨hal-04454043⟩
- Alexandre Bonvoisin, Clément Quinton, Romain Rouvoy. Understanding the Performance-Energy Tradeoffs of Object-Relational Mapping Frameworks. SANER'24 - 31th IEEE International Conference on Software Analysis, Evolution and Reengineering, Mar 2024, Rovaniemi, Finland. pp.11. ⟨hal-04401643v2⟩
Preprints, Working Papers
- Francesco Diana, Othmane Marfoq, Chuan Xu, Giovanni Neglia, Frédéric Giroire, et al.. Attribute Inference Attacks for Federated Regression Tasks. 2024. ⟨hal-04792394⟩
- Danilo Carastan-Santos, Georges da Costa, Igor Fontana de Nardin, Millian Poquet, Krzysztof Rzadca, et al.. Scheduling with lightweight predictions in power-constrained HPC platforms. 2024. ⟨hal-04747713⟩
- Fabiano Lorusso, Frédéric Giroire, Joanna Moulierac, Guillaume Urvoy-Keller. Enhancing Energy Efficient Task Caching and Offloading in Multi-Access Edge Computing. 2024. ⟨hal-04721135⟩
- Davide Ferre’, Cinzia Di Giusto, Etienne Lozes, Nicolas Nisse. Weakly synchronous systems with three machines are Turing powerful. 2024. ⟨hal-04716163v2⟩
- Davide Ferre’, Cinzia Di Giusto, Etienne Lozes, Nicolas Nisse. Weakly synchronous systems with three machines are Turing powerful *. 2024. ⟨hal-04727303⟩
Theses
- Pierre Jacquet. Enhancing IaaS Consolidation with Resource Oversubscription. Systems and Control [cs.SY]. Université de Lille, 2024. English. ⟨NNT : ⟩. ⟨tel-04685771⟩
Other projects